Preferences
Related Series
Related Segments
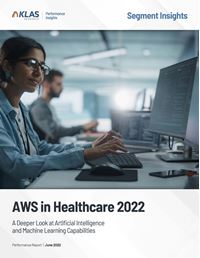
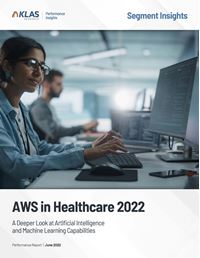
AWS in Healthcare 2022
A Deeper Look at Artificial Intelligence and Machine Learning Capabilities
Excited by the potential time and money savings, many healthcare organizations want a deeper understanding of what artificial intelligence (AI) and machine learning (ML) technology from public cloud providers could do for them. This research, a follow-up to KLAS’ 2021 report on Amazon Web Services (AWS), examines the experiences of 13 payer and provider organizations using AWS AI/ML offerings. This study is meant to help organizations considering AWS understand what technologies are being used, the overall customer experience, and the impact of these offerings. This report also shares AWS clients’ insights into their selection process and their perceptions of Google Cloud Platform (GCP) and Microsoft Cloud (both of which will be the focus of future reports). To learn how KLAS defines AI and ML, see Healthcare AI CHIME Edition 2019: Actualizing the Potential of Artificial Intelligence.
Operational Optimization Most Widely Deployed Use Case; Forward-Looking Energy Focused on Clinical Impact
Across the healthcare industry, payers and provider organizations are increasingly turning to AI- and ML-enabled technologies to streamline and optimize processes. Common operational use cases adopted by provider organizations include incorporating consent forms in the EMR, improving patient flow in the OR by gathering needed PHI before surgery, converting unstructured patient notes into structured formats, accelerating access to needed PHI, and automatically documenting discussions between patients and physicians. Payers use AWS solutions to automatically extract and convert faxed information from physician offices for prior authorizations and to convert handwritten documents such as grievances, appeals, and member enrollment forms. Both groups are leveraging Amazon AI/ML tools like Comprehend Medical and SageMaker to better understand the consumer experience and to engage patients or members by connecting them to care guides. Other solutions, such as Lex, are used to proactively tackle care management by connecting high-risk cohorts with their doctors to ensure they get emotional support, reminders for care-gap closures, and even COVID-19 screenings.
Reduced Manual Processes & Time Savings Are Key Outcomes
Time savings are a core outcome identified by many respondents. Examples include reducing the time to extract and convert handwritten information from days to hours, reducing after hours physician documentation, and less administrative time spent entering consent forms into the EMR. When it comes to the patient/member experience, both payers and providers say leveraging multiple AWS tools allows for greater continuity of care. These tools have streamlined access to care, helped members connect with healthcare staff that have helped them previously, and provided sentiment analysis of conversations to support real-time experience improvement. The organizations that report seeing process improvements use Comprehend Medical, Lex, SageMaker, Textract, and Transcribe Medical, in addition to other infrastructure and platform services. Organizations that have seen improvements in the patient experience note the use of Comprehend Medical, HealthLake, Lex, SageMaker, and Textract.
Beyond Technology, Strong Service & Support Drive Perceptions of AWS as a Partner
Customers consistently describe AWS as a proactive, reliable partner who is committed to client success. Respondents appreciate the vendor’s willingness to meet with all members of an organization’s team, not just high-level decision-makers, to help them understand how to be successful. Ongoing support personnel are accessible, deeply know the customer’s solutions, and encourage regular communication and touch points. Several respondents say that their success with the tools has come directly as a result of the focus and attention they receive from AWS. These positive experiences drive a high level of customer loyalty. Most respondents are excited to see what else AWS can offer in the future and would recommend AWS AI/ML capabilities to their peers.
To Support Usability, Customers Encourage AWS to Make Training a Stronger Focus
When organizations have a positive experience with onboarding, they report better ease of use and describe the platform as intuitive; in contrast, weaker training and onboarding is tied to lower ease-of-use ratings. In general, customers encourage potential clients to purchase time with AWS’ tech team to develop the internal technical expertise necessary to be successful. Organizations who have received this training say it is useful but is not well advertised to clients as an option. AWS could improve client success by better communicating that this training is available and important, along with ensuring their training specialists have a strong understanding of various healthcare audiences.
AWS Customers’ View of the Market: Microsoft Cloud a Viable Competitor, Less Enthusiasm for Google Cloud Platform
For this study, KLAS asked AWS customers about their platform-selection process and considerations. Many say they selected AWS because it was the most mature platform at the time. Other common reasons for selection are name recognition, Amazon’s reputation for creating strong and highly integrated consumer-facing applications, high system reliability and performance, and strong security and privacy.
These AWS customers also shared their perceptions of and experiences with Google Cloud Platform (GCP) and Microsoft Cloud during the selection process. Upcoming KLAS reports will share feedback from Google and Microsoft customers, in addition to these organizations’ perceptions of AWS.
About This Report
Each year, KLAS interviews thousands of healthcare professionals about the IT solutions and services their organizations use. As cloud offerings cover both software and services, this report draws on a hybrid set of quantitative evaluation questions from KLAS’ standard software and services evaluations, which are composed of numeric ratings questions and yes/no questions. KLAS also created a supplemental evaluation to delve deeper into several questions specific to AWS’ AI/ML offerings.
Healthcare organizations interviewed for this report come from an AWS-provided list of “leading customers”; as a result, this research is meant to convey insights on only a subset of the healthcare cloud market and is not representative of the market or the AWS customer base as a whole. The number of unique responding organizations is given in the chart below.
Sample Sizes
Unless otherwise noted, sample sizes displayed throughout this report (e.g., n=16) represent the total number of unique customer organizations interviewed. However, it should be noted that to allow for the representation of differing perspectives within any one customer organization, samples may include surveys from different individuals at the same organization. The table below shows the total number of unique organizations interviewed as well as the total number of individual respondents.
Some respondents choose not to answer particular questions, meaning the sample size can change from question to question. When the number of unique organization responses for a particular question is less than 15, the score for that question is marked with an asterisk (*) or otherwise designated as “limited data.” If the sample size is less than 6, no score is shown. Note that when a vendor has a low number of reporting sites, the possibility exists for KLAS scores to change significantly as new surveys are collected.
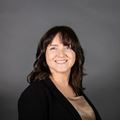
Writer
Amanda Wind
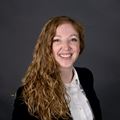
Designer
Madison Moniz
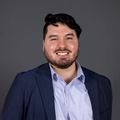
Project Manager
Joel Sanchez
This material is copyrighted. Any organization gaining unauthorized access to this report will be liable to compensate KLAS for the full retail price. Please see the KLAS DATA USE POLICY for information regarding use of this report. © 2025 KLAS Research, LLC. All Rights Reserved. NOTE: Performance scores may change significantly when including newly interviewed provider organizations, especially when added to a smaller sample size like in emerging markets with a small number of live clients. The findings presented are not meant to be conclusive data for an entire client base.