Preferences
Related Series
Related Segments
Related Articles


Healthcare EMR AI 2020
A Closer Look at EMR Offerings
Previous KLAS research on artificial intelligence (AI) in healthcare examined customer satisfaction with AI-focused vendors. However, as provider organizations develop go-forward AI strategies, they want to know: What capabilities are available from the solution that represents their organization’s largest HIT investment—their EMR? To answer this question, this report investigates what EMR vendors are doing in the world of AI.
KLAS defines healthcare AI software as software that provides machine learning (ML) or natural language processing (NLP) capabilities for healthcare-related clinical, operational, or financial areas.
AI Adoption—From CHIME’s 2019 HealthCare’s Most Wired Report
As part of CHIME’s recent Most Wired survey, 483 provider organizations described their progress in adopting various types of AI solutions.
EMR VENDORS' AI STATUS:
ALLSCRIPTS, CERNER, EPIC & MEDITECH
What’s Live Today, and What’s Planned for the Future?
Allscripts
Has an internal research/data science team and has been using client data for some internal AI/ML projects for the last several years. Nearing the phase when they will be able to start productizing an offering. For ML, has been working with a partner customer on a model to predict acute kidney injury. Also developing ambient AI to reduce the clinician workload.
Cerner HealtheDataLab
Fewer than 10 customers (most in pilot phase); two shared feedback for this research. Product is ML development platform customers can use to build and deploy their own algorithms; does not include a stock catalog of prebuilt ML models. Data is fed through HealtheIntent. New partnership with Amazon Web Services (AWS) allows platform to be cloud hosted and bring in data (including non-Cerner data) from a variety of sources. Other AI applications within the EMR include:
- Charge Assist (generally available; helps with accurate coding for E&M codes)
- Chart Assist (generally available; helps with documentation improvement)
- Chart Search (generally available; NLP-driven search engine spanning EMR and PHM records)
- Virtual Scribe (alpha phase; transcribes clinician/patient conversations)
- Voice Assist (proof-of-concept phase; enables completion of certain tasks via voice command)
Epic Cognitive Computing Platform
Most widely adopted EMR AI solution; only EMR with enough live customers for satisfaction data to be shared (see satisfaction section below). Offers NLP capabilities; has both prebuilt ML models as well as an ML development platform.
- Most Epic AI customers are “out-of-the-box” users, meaning they are using prebuilt ML models from Epic’s Model Library, available to Cogito customers. They also receive technical support for testing, validation, and deployment.
- In addition to using prebuilt models, customers with more advanced AI needs or capabilities can license Cognitive Computing Developer Platform and engage Epic’s services to customize models to local populations (through cloud or on-premises option) and build/deploy their own algorithms, incorporating non-Epic data if needed (vast majority of validated use cases involve only Epic data).
- Clinical voice assistant (named Hey Epic!) currently available; ambient listening is in development.
MEDITECH
Focus is on AI capabilities outside of developing an AI platform or providing prebuilt ML models. Two main areas of focus are (1) virtual assistant to enable voice navigation within patient charts (plans to pilot in Q3 of 2020); and (2) ambient listening (mostly in research phase). First planned use case for ambient listening is the ability through an app or device to turn clinician/patient conversation into structured data to create a history and physical or a consultation or progress note.
USE CASES: CERNER & EPIC
To Date, Cerner & Epic the Only EMR Vendors with Live AI Solutions
The two interviewed Cerner customers (both in pilot) populate the AI platform with data from HealtheIntent and leverage HealtheDataLab to build and run custom ML models that meet their localized business needs. This requires the expertise of internal AI resources—such as data scientists, analysts, and other clinical support resources—and both clients recommend having such resources as a best practice for peers. While it is up to customers to operationalize the models, both interviewed clients say Cerner has been hands-on and responsive in helping set up the models. One customer is a multi-tenant AWS client and reports a desire for greater flexibility. Both customers report achieving tangible outcomes using the AI models built with HealtheDataLab. Most reported use cases are in clinical areas and include utilization prediction, A1c prediction, readmission prediction, and heart failure prediction.
Epic’s Cognitive Computing Platform is chosen for a number of reasons:
- It enables Epic EMR customers to access AI capabilities without having to engage another vendor. Some customers do also partner with other AI vendors for tools or models that Epic doesn’t offer or for projects that use non-Epic data (though few customers pull in non-Epic data; those that do report heavy lifting on their part and recommend in-house analytics expertise).
- If Epic data is used, the ML models are fairly easy to deploy and integrate into workflows. Customers must still solve the operational part of the equation themselves, but the platform minimizes the amount of technical work needed. The workflow integration aids in clinician adoption and is one of the platform’s biggest advantages. Epic customers use Epic’s AI models most heavily in clinical areas, with readmission and sepsis prediction being the most frequently reported use cases. The operational use cases mostly involve bed planning and no-show prediction.
“Epic began to put a lot of time and effort into the predictive models a few years ago. When determining our AI strategy, part of the discussion was whether we should stick with Epic rather than having multiple vendors whose products weren’t necessarily aligned. I have pushed to make sure that our analytics come from one system as much as possible so that doctors don’t have to be logging in to other systems or looking at reports outside of Epic. Unless third-party systems are really fully integrated with Epic, then we get clunky workflows.”
—CMIO, small health system
“We have Epic, we have third-party systems, and we have our own AI team. With varying degrees of success, we have incorporated elements from each of those three buckets into our operations. Epic has been the easiest. One third-party solution didn’t really work out. The other is working out. But integrating the third-party solutions into Epic required a lot of work. It is certainly easier to use native Epic functionality.”
—CMIO, academic health system
CUSTOMER SATISFACTION: EPIC
As noted above, Epic is the only EMR vendor with enough live customers for customer satisfaction data to be shared. Thus, the remainder of this report will focus on helping Epic’s EMR customers understand the user experience of peers already leveraging Cognitive Computing Platform.
Epic AI Still Maturing but a Good Option for EMR Customers Prepared to Operationalize Models and Drive Outcomes Themselves
Customers are still relatively new to Cognitive Computing Platform, having used it on average a little less than two years. Although customers don’t view the solution as the most advanced AI product in the market, they say it is evolving and improving quickly, much the way other Epic products have. Some interviewed customers feel Epic has also improved implementations and support in response to customer feedback, and all plan to keep the solution long term.
Cognitive Computing Platform: Epic Customer Experience Compared to the AI Market
“Epic is very responsive to us. Every time we want to reach out to them and need help, they are great. It is hard to say how much of a role they have played in us achieving outcomes. Rather than the tool, how people are deploying interventions is what makes the difference. Epic is not quite there with integrating their models, especially together. AI has nothing to do with measuring whether workflows are being complied to and consistent. We could have the best predictive model in the world, but if we are not solving for those other things, we just have great technology in a box.”
—Assistant vice president, data analytics
Customers rate Epic below the AI market average for training, proactive service, executive involvement, and tangible outcomes, reporting less partnership compared to AI-focused vendors and to what Epic provides for other product lines.
- On the positive side, Epic is responsive to customer questions, provides some training, and helps get the ML models up and running. Additionally, customers report achieving outcomes pretty quickly, especially advanced customers who have integrated the models into the clinician workflow. Reported outcomes include decreased readmissions, decreased mortality rate, and decreased number of rapid-response-team initiations.
- However, whereas most of the AI-focused vendors are noted for their collaboration in helping customers achieve ROI and desired outcomes, Epic’s support is focused mainly on how to set up the models. Customers would like Epic to be more proactive in sharing best practices for training the models, deploying them effectively, and prioritizing which models to use instead of leaving customers to figure out how to leverage the technology on their own.
Epic Out-of-the-Box Users vs. Advanced Users: Biggest Differences Seen in Relationships and Value
Both out-of-the-box users and more advanced customers say Epic has done a good job of embedding explainable AI, creating transparency for and trust in the platform’s predictions. Customer feedback from the two groups differs most in their perceptions of value and the vendor/client relationship.
Value: The biggest difference lies in how well the two groups are able to demonstrate outcomes. Operationalizing models, embedding them in the workflow, and driving adoption all fall largely on customers’ shoulders. Having more AI experience and more internal talent to lead these efforts, advanced customers are more likely to report tangible outcomes. Lacking additional guidance from Epic (which they are accustomed to receiving for other Epic products), out-of-the-box customers who don’t have needed internal resources report lower perceptions of value.
Relationships: Both groups receive good support for validating and setting up models and note Epic’s responsiveness and willingness to help. A few out-of-the-box customers report less consistent technical and operational/workflow expertise from Epic’s support staff.
“Epic has a host of models that are available to us, but we haven’t turned all of them on. Most of our challenges are cultural. The Epic model is something that we are not used to looking at operationally. The data is great, but I don’t know what I am supposed to do with it. We need an appropriate workflow to address that issue. . . . I would definitely like to turn on more of Epic’s predictive models, but I am hesitant. With our structure, . . . the biggest challenge is deciding who will consume and act upon the data when we turn on a certain predictive model.”
—Director of healthcare analytics (out-of-the-box organization)
Are the Advanced Capabilities Worth It?
So—should Epic EMR customers invest in the additional capabilities beyond the out-of-the-box ML models? Current users say yes, provided an organization has already reached a certain level of AI maturity, including (1) a good internal data science team or interdisciplinary team capable of validating and managing the models, (2) the appetite to build/deploy their own models and customize/tweak Epic’s prebuilt models, and (3) a great process for operationalizing the new models Epic continues to release.
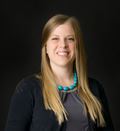
Writer
Elizabeth Pew

Designer
Jess Wallace-Simpson
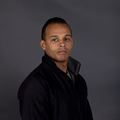
Project Manager
Robert Ellis
This material is copyrighted. Any organization gaining unauthorized access to this report will be liable to compensate KLAS for the full retail price. Please see the KLAS DATA USE POLICY for information regarding use of this report. © 2024 KLAS Research, LLC. All Rights Reserved. NOTE: Performance scores may change significantly when including newly interviewed provider organizations, especially when added to a smaller sample size like in emerging markets with a small number of live clients. The findings presented are not meant to be conclusive data for an entire client base.