Preferences
Related Series
Related Segments
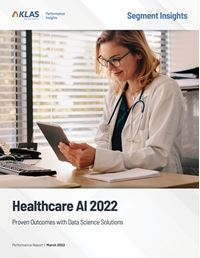
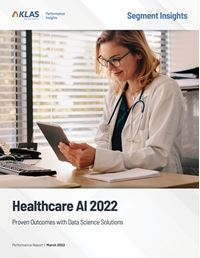
Healthcare AI 2022
Proven Outcomes with Data Science Solutions
The potential for artificial intelligence (AI) to transform healthcare has been both championed and challenged. In 2019, KLAS published our first research on healthcare AI data science solutions, exploring healthcare organizations’ clinical, financial, and operational use cases and the early outcomes they were achieving. An update to that research, this report examines how outcomes and customer satisfaction have evolved in the years since. Though progress has been somewhat hamstrung by the financial and operational constraints of COVID-19, many of the organizations interviewed for this research have found results by focusing on the right problems.
This report includes two types of data: (1) customer satisfaction ratings for several key performance metrics, and (2) case studies from individual organizations detailing their use cases, outcomes, and lessons learned. Summaries of the case studies are shared on the following pages. The complete case studies can be found in the full report.
Struggling Jvion Customers Leaving Due to Unmet Outcomes and Financial Constraints
Citing pandemic-related financial constraints and a lack of worthwhile outcomes, a high number of Jvion customers have left the previous market share leader. KLAS has validated that of the 38 clients Jvion reported to KLAS in 2019, at least 12 no longer use the product (Jvion has not shared an updated client list). Organizations that are staying say that to make the system impactful, they need more proactive support to promote adoption and turn the models’ data into actionable insights. They also want the prescriptive outputs to better integrate into EMR workflows. A couple of respondents say progress is finally being made following major leadership changes over the last couple of years.
ClosedLoop.ai Provides Top-Shelf Experience; Satisfaction with Health Catalyst Surges Following Increase in Prescriptive Guidance
Customers are highly satisfied with ClosedLoop.ai, who is used by both payer and provider organizations to improve risk scores, decrease readmissions, close care gaps, and reduce claims denials. Reported strengths include strong implementations and consultative partnering and guidance. The vendor excels at helping customers identify the root problems they want to solve and then find models that will deliver the desired results. Additionally, ClosedLoop.ai is highly involved in change management, sharing best practices, and helping organizations implement them; this leads to better physician adoption and thus better outcomes (see next chart). One potential hindrance is the need for technical resources within the customer organization to support the solution and monitor the models.
Customer satisfaction with Health Catalyst has jumped sharply over the last year as the company has improved at digging into customers’ data and providing prescriptive guidance as to where they should focus their AI efforts. Customers report that this guidance enables them to focus on the right populations and problems. They speak highly of the vendor’s expertise and willingness to help them achieve their goals. Newer AI customers in particular have experienced close partnering during implementation.
Ease-of-Use Challenges Can Hinder Outcomes for Cerner and Epic Customers
Though Cerner and Epic EMR users often turn to their enterprise vendors for AI capabilities, ease of use is a concern and can hinder outcomes. Epic customers can choose a limited number of prebuilt models from a broad library at no additional cost. These users represent the bulk of Epic’s AI customer base, and most use the models for clinical use cases. A growing number of customers are starting to also license Cognitive Computing Developer Platform, which allows for custom model deployment. Customers feel the prebuilt models are generally well integrated into their workflows. However, many report that testing these models and getting them to a state where they are delivering outcomes requires a significantly larger lift than expected. Epic’s customer support is typically a strength, and many respondents say it is easy to get ahold of the support resources. Feedback on training and proactive guidance is mixed—some describe the documentation as strong, and others feel it lacks needed information. Customers must pay for additional prebuilt models (beyond those that are free), leading 25% of respondents to mention nickel-and-diming as a frustration. Despite a few challenges, customers are optimistic about Epic’s direction and believe the vendor will help them achieve their goals.
Adoption of Cerner’s AI solutions has recently grown. Users of the prebuilt ML models (referred to as Managed ML Models) report more success than those who develop their own models (via HealtheDataLab). Regardless of which solution they are using, many respondents report difficulty getting the models up and running. Users of the prebuilt models are more likely to report guidance from Cerner on how to utilize the data, and they are also more likely to report achieving desired outcomes (e.g., reduced readmissions and improved care management). Customers that choose to build their own models struggle more and say they would benefit from additional training, documentation, and guidance to help them achieve desired results.
Case Study Summaries
To more deeply explore the current state of healthcare AI, KLAS interviewed two of each vendor’s deepest AI adopters regarding their AI use cases and the outcomes being achieved. Summaries of the case studies are shared below. The complete case studies can be found in the full report. These case studies are meant to both showcase specific vendor capabilities and encourage healthcare organizations that have not harnessed AI technology to consider how it could benefit their organizations.
Summary of case studies
Case Study #1: Encompass Health
Preventing Readmission in Post–Acute Care Population: Using two models that predict likelihood of hospital readmission during and after a post–acute care stay, Encompass Health has decreased readmissions and seen financial benefits. Getting buy-in and adoption from those who would use the information was key to success.
Case Study #2: Northern Light Health
Using a Command Center to Improve Hospital Operations: Northern Light Health’s command center has utilized AI algorithms to improve patient flow management and real-time visibility to help with decision-making. Building trust and encouraging adoption were key areas of focus.
Case Study #1: Healthfirst
Improving Care Plan Adherence and Reducing Readmission Risk: Healthfirst uses their models to identify and then reach out to patients at risk for readmission or for care plan noncompliance, employing different reach-out methods based on the patient’s risk level.
Case Study #2: Medical Home Network (MHN)
Automated Identification of Care Management Candidates: To optimize their limited number of care management resources, Medical Home Network uses a cost-of-care AI model to identify the top 4% of patients who should be prioritized for care management. Another model helps them identify patients at risk for readmission.
Case Study #1: OhioHealth
Achieving Clinical Benefits with Prebuilt Models: OhioHealth has implemented 17 AI models, 10 of which are prebuilt models from Epic. Results include improved sepsis monitoring and reduced length of stay. They recommend engaging key stakeholders and creating a long-term maintenance plan.
Case Study #2: UW Health
Improving Organization Challenges with a Combination of Prebuilt and Custom Models: UW Health utilizes prebuilt models to help them reduce readmissions and sepsis rates and leverages custom models to prevent falls and intervene earlier in sepsis cases. They recommend doing your homework to decide when it would be best to use prebuilt vs. custom models.
Case Study #1: ChristianaCare
Enabling Better Identification of Heart Failure Patients at Risk for Hospital Readmission: By putting data from their AI models directly in their care managers’ workflows, ChristianaCare enables these resources to make better decisions as to which heart failure patients might be at risk for hospital readmission. Using the models has led to reduced readmissions (from well over 20% down to about 16%) and positive shared savings.
Case Study #2: INTEGRIS Health
Using Augmented Intelligence to Guide Strategic Initiatives and Determine Areas of Focus: INTEGRIS Health uses AI to establish strategic benchmarks, set realistic stretch goals throughout their organization, and then measure improvement. They put strong emphasis on using the data to set the right goals in the right areas.
Case Study #1: SCAN Health Plan
Targeting Improved Care Management for At-Risk Seniors: SCAN Health Plan uses AI to focus on the at-risk senior members who could most benefit from care management. They have improved their identification of at-risk seniors by 20%–30%.
Case Study #2: St. Luke’s University Health Network
Optimizing Care Management Efforts by Assessing Population Needs: To ensure they are focusing their care management efforts on the right patients, St. Luke’s University Health Network created a dashboard for HEDIS metrics to help them identify high-risk patients with conditions such as diabetes or hypertension.
Case Study #1: Geisinger Health System
Using AI to Identify and Target Patients Most Likely to Need Colorectal Cancer Screening: Geisinger Health System uses AI algorithms to identify the patients most at risk for colorectal cancer and to encourage them to receive screenings. They estimate that the program saves 5–6 lives per year.
Case Study #2: Kaiser Foundation Health Plan of the Northwest
Promoting Preventive Care for Members Most at Risk for Hospitalization from Flu or COVID-19: Kaiser Foundation Health Plan of the Northwest utilizes AI to identify the members most at-risk for hospitalization from flu or COVID-19. They then use these lists to contact members and encourage preventive care.
Note: Jvion declined to provide candidates for case studies.
Recommendations from Successful Organizations
Preimplementation
- Analytics solutions are foundational to AI and often need to be implemented before predictive/prescriptive models.
- Identify the problem you are trying to solve. Then determine whether AI is the right solution for the problem.
- Set meaningful, clearly articulated goals for your use cases.
- An out-of-the-box solution is a great place to start, but the models will require examination before the predictions or prescriptive output can be implemented.
- For each use case, collaborate and get buy-in across teams before, during, and after the implementation; create an inclusive governance structure that represents all stakeholder groups and plan carefully for change management.
“I often see people who want to throw AI at everything, but it isn’t the right solution for every problem. We run about 10 models. We get a very high ROI from all of those models because we were very prescriptive in determining which scenarios we could use AI in to really deliver value. Good opportunities for AI are scenarios that require a lot of data. People should focus on use cases where they need AI to learn a lot of data and predict various conditions. Not everything is a good use case for AI.” —CIO
Implementation
- Don’t get hung up on perfecting the incoming data. The machine learning needs to happen with your data as it really is.
- Plan for the model testing to take more time and resources than expected.
- Don’t spend too much time trying to perfect a model. Models that are “good enough” can still drive significant outcomes.
- Figuring out how to operationalize the model (i.e., defining the intervention) often requires more work than the actual build.
- Foster buy-in and adoption by helping end users understand the models and how the data is generated.
“The biggest challenge with AI is operationalizing the models. We could create models left and right all day long, but they don’t mean anything until they are put into operation and can make a difference in decision-making.” —VP
Change Management & Long-Term Success
- Drive long-term outcomes and prevent model drift by making sure your team is educated and ready to support the models, which will need continued iteration.
- For long-term success, integrate the models into processes and systems, especially the EMR.
“I would tell people to go in with a pretty open mind because there is more to learn than people think. People are probably better off going in with an open mind about the possibilities rather than demanding x, y, and z. Developing the models is a very iterative process.” —VP
About This Report
The data in this report comes from two sources: (1) case study interviews with two of each vendor’s deepest AI adopters and (2) KLAS performance data.
Case Study Interviews
To more deeply explore the current state of healthcare AI, we interviewed two of each vendor’s deepest AI adopters regarding their AI use cases and the outcomes being achieved. Helpful insights from each of these interviews can be found in the full report.
KLAS Performance Data
Each year, KLAS interviews thousands of healthcare professionals about the IT solutions and services their organizations use. For this report, interviews were conducted over the last 12 months using KLAS’ standard quantitative evaluation for healthcare software, which is composed of 16 numeric ratings questions and 4 yes/no questions, all weighted equally. Combined, the ratings for these questions make up the overall performance score, which is measured on a 100-point scale. The questions are organized into six customer experience pillars—culture, loyalty, operations, product, relationship, and value.
Sample Sizes
Unless otherwise noted, sample sizes displayed throughout this report (e.g., n=16) represent the total number of unique customer organizations interviewed for a given vendor or solution. However, it should be noted that to allow for the representation of differing perspectives within any one customer organization, samples may include surveys from different individuals at the same organization.
The table to the left shows the total number of unique organizations interviewed for each vendor or solution as well as the total number of individual respondents.
Some respondents choose not to answer particular questions, meaning the sample size for any given vendor or solution can change from question to question. When the number of unique organization responses for a particular question is less than 15, the score for that question is marked with an asterisk (*) or otherwise designated as “limited data.” If the sample size is less than 6, no score is shown. Note that when a vendor has a low number of reporting sites, the possibility exists for KLAS scores to change significantly as new surveys are collected.
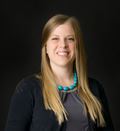
Writer
Elizabeth Pew
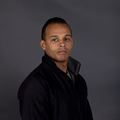
Project Manager
Robert Ellis
This material is copyrighted. Any organization gaining unauthorized access to this report will be liable to compensate KLAS for the full retail price. Please see the KLAS DATA USE POLICY for information regarding use of this report. © 2025 KLAS Research, LLC. All Rights Reserved. NOTE: Performance scores may change significantly when including newly interviewed provider organizations, especially when added to a smaller sample size like in emerging markets with a small number of live clients. The findings presented are not meant to be conclusive data for an entire client base.