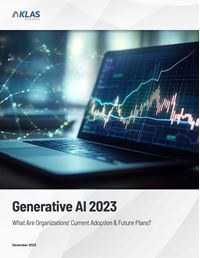
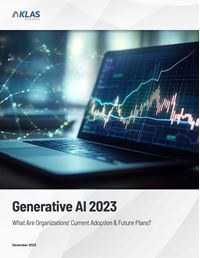
Generative AI 2023
What Are Organizations’ Current Adoption & Future Plans?
Across multiple industries, interest in generative AI is spiking, especially as more easy-to-access solutions enter the market. Unsurprisingly, healthcare organizations are among those interested in generative AI’s potential; however, many have concerns about its reliability and security and want to understand its true capabilities amid all the buzz. To explore some of these questions and concerns, this report—KLAS’ first to focus on generative AI—draws from the perspectives of 66 healthcare executives, sharing their current adoption, future plans, and current challenges regarding generative AI. KLAS intends to publish further general AI insights with the Center for Connected Medicine (CCM) in early 2024.
If you don't have a login, getting started is easy.
Generative AI Definition
Generative AI refers to AI that can create text, images, or other media by learning from existing data and generating new content with similar characteristics. It utilizes generative models to understand patterns and structures in the training data, enabling it to produce novel and coherent outputs.
Despite Newness, Generative AI Solutions Are Being Adopted, Mostly by Larger Organizations
In general, the number of organizations adopting generative AI solutions is relatively small, and most who have adopted say it is still too early to report outcomes. Though adoption is particularly low among smaller organizations, many larger organizations and other entities like payers have already embraced various generative AI tools from EHR vendors (e.g., Epic) as well as from Google, Nuance, and OpenAI. The higher adoption among larger organizations is likely attributed to their higher number of resources, which enable them to adopt generative AI solutions more swiftly. In addition, larger organizations often have data more readily available and employ data scientists and specialists who can effectively develop, implement, and drive outcomes with these solutions.
Most Organizations Are Likely to Purchase/Implement Generative AI in Coming Year
Although only 25% of interviewed respondents have implemented generative AI solutions, 58% say their organization is likely to implement or purchase a solution within the next year. As previously stated, larger organizations are more inclined to invest in a solution than smaller organizations. Those who plan to invest are primarily motivated by the desire to enhance efficiency. Due to the numerous challenges organizations face, including staffing and financial constraints, it is critical for them to experiment with various methods that can drive efficiency across their enterprise.
“The release of ChatGPT sparked a conversation around how we are going to utilize AI in our organization. It has led me to pay a lot more attention to where we are using AI already as a system and to think strategically about how we can use AI to help us do our jobs.” —CMIO
Organizations Hope Generative AI Will Increase Efficiency around Documentation, Patient Communication & Workflow
Respondents are excited about the opportunities generative AI can create; however, many don’t yet know where they will use the AI in their organizations. Respondents who have a strategy are most likely to purchase generative AI solutions to increase efficiency around documentation, patient communication, and workflow. Documentation plays an important role in ensuring effective patient care but is often time consuming and riddled with errors. By leveraging generative AI, respondents hope to automate the process of generating clinical notes, summaries, and reports based on patient data, thereby saving a significant amount of time. Further, generative AI can play a pivotal role in facilitating effective, personalized patient communication by delivering tailored, timely information to patients. These solutions can also address patient queries, provide medication reminders, and offer general health advice, resulting in improved patient engagement and adherence to treatment plans. Additionally, generative AI has the potential to streamline an organization’s workflows and operations by automating documentation, prewriting notes, templating emails, etc. As more organizations start leveraging generative AI, the solutions will need to drive hoped-for outcomes and prove a strong ROI to stay relevant and viable in the market long term.
Accuracy/Reliability Is Far and Away the Biggest Challenge of Using Generative AI Solutions
Respondents report several challenges associated with generative AI; the top three challenges are accuracy/reliability, cost/ROI, and security/privacy. Accuracy/reliability is mentioned more than twice as much as the other challenges. Accuracy is critical when using AI in healthcare, and respondents worry that inaccuracies, bias, and AI hallucinations would negatively impact patients and decisions. It is also important for solutions to be proven reliable by users. Cost/ROI is the second most-mentioned challenge. Implementing and maintaining AI infrastructure—including hardware, software, and skilled personnel—can be expensive. Moreover, the initial investment may not yield immediate returns, making it essential for organizations to carefully assess the long-term benefits and potential cost savings of generative AI. This assessment allows organizations to secure funding and support for ongoing AI initiatives; however, proving that generative AI is driving outcomes can be difficult. Security/privacy is another frequently mentioned challenge. Patient data is highly sensitive and subject to strict regulations (e.g., HIPAA), and it is paramount for organizations to protect patient privacy and ensure data security throughout the AI workflow. Organizations must implement robust encryption, access controls, and anonymization techniques to safeguard patient information and maintain compliance with regulatory requirements.
Want More KLAS Data on AI?
Click the following report links for more KLAS insights:
About This Report
This report is a perception study designed to help readers understand how healthcare organizations are using generative AI today, what their future plans are, and what challenges they are experiencing today. To gather these perspectives, KLAS created a supplemental evaluation and asked 66 healthcare executives the following questions that are specific to generative AI:
- Is your organization currently using solutions with generative AI, and if so, what vendors are you using?
- Over the next 12 months, how likely is your organization to purchase/implement a solution with generative AI?
- What types of solutions with generative AI does your organization plan to purchase/implement?
- What are the biggest opportunities for generative AI in healthcare?
- What are the biggest challenges of generative AI in healthcare?
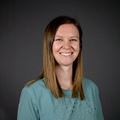
Writer
Sarah Hanson
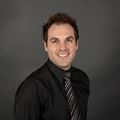
Designer
Bronson Allgood
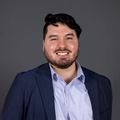
Project Manager
Joel Sanchez
This material is copyrighted. Any organization gaining unauthorized access to this report will be liable to compensate KLAS for the full retail price. Please see the KLAS DATA USE POLICY for information regarding use of this report. © 2024 KLAS Research, LLC. All Rights Reserved. NOTE: Performance scores may change significantly when including newly interviewed provider organizations, especially when added to a smaller sample size like in emerging markets with a small number of live clients. The findings presented are not meant to be conclusive data for an entire client base.