Preferences
Related Series
Related Segments
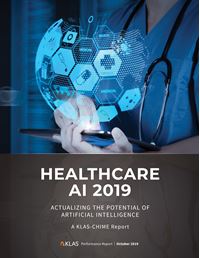
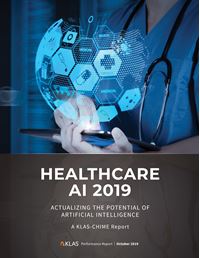
Healthcare AI 2019
Actualizing the Potential of Artificial Intelligence
Artificial intelligence (AI) has been a buzzword in healthcare for several years, but how real is it? Are healthcare organizations adopting AI? How are they using it and what outcomes are they seeing? Based on interviews with 57 trailblazing healthcare organizations, this report—KLAS’ first on this emerging area (specifically machine learning and NLP)—consists of two types of findings: (1) an AI primer with insights on needed AI capabilities, common challenges, and best-practice advice; and (2) early findings about what outcomes healthcare organizations are achieving with AI technology and how well their vendors drive value. Overall, vendor performance in the AI space is high (compared to other software market segments measured by KLAS).
Across the technology and healthcare industries, definitions of AI vary widely; KLAS’ working definition of AI was created for use in research regarding customer satisfaction with AI technology and was formed based on advice from many leading health systems and vendors.
INDUSTRY INSIGHTS AND CONTEXT
A KLAS-CHIME Report†
† Research conducted in partnership with CHIME. Participating provider organizations were engaged through CHIME outreach to members (for this report and for the 2019 CHIME HealthCare’s Most Wired survey) and/or KLAS’ traditional, client list–based outreach.
KLAS PERFORMANCE INSIGHTS
Because healthcare AI is such a new market, only one of the six measured vendors—Jvion—has enough evaluations (at least 15) to be considered fully rated. Findings on all other vendors are based on limited data (6–15 evaluations), and in these cases, it is possible KLAS scores may change as new surveys are collected; notably, KLAS spoke with a large percentage of vendors’ live customers. See the Expanded Insights section for details on KLAS’ data thresholds.
DataRobot and KenSci Drive Customer Success with Differentiating Service
All interviewed DataRobot clients tout the vendor’s customer-focused partnership approach, which is unique in healthcare IT. Support resources ensure high-performing models and successful deployments. Many clients say DataRobot’s robust training and certification process helps internal teams without a data science background successfully build models. The vendor’s guidance shows their investment in customer success and relationships. Many data-related tasks are left up to the client, though DataRobot data scientists are willing to provide support. Clinical expertise is also lacking, as are prebuilt healthcare models.
KenSci collaborates closely with clients, providing data science expertise, listening to constructive feedback from customers, and incorporating changes. This partnership drives very high customer satisfaction. All respondents describe KenSci as an engaged partner. Most customers acknowledge KenSci has work to do to mature their platform; the collaboration between KenSci and their customers to develop and learn requires significant time to produce value.
Top-of-Mind IBM Watson Health Starting to Turn the Corner in Performance, Still Has Room to Improve in Providing Value
IBM Watson Health, one of the most highly discussed AI technologies in healthcare, is unique among vendors measured in this report because their AI-enabled point solution (the type of solution this report is focused on) represents a subset of their AI platform, a much broader analytics and services offering. Historically, development partners felt the IBM Watson Health solution took a long time to learn and did not achieve desired outcomes. Today, most validated customers use Watson’s ML and NLP technology with a clinical focus and fairly narrow scope. Most IBM customers are happy with the product and IBM’s collaborative approach—e.g., training users on data science, bringing in clinical and data science experts, and being responsive and available to address client issues or questions. When IBM doesn’t take a hands-on approach to helping their customers, organizations struggle to progress and achieve desired outcomes. In terms of the technology, Watson AI has the most comprehensive store of indexed medical publications,
which is kept up to date. This offering is unique and is used for highly valuable use cases, such as medical research, genomics analysis, and education for cancer patients. A couple of customers question whether the product, while helpful, is worth the price tag long term. Two of the seven interviewed IBM customers report nickel-and-diming, the highest percentage among measured vendors.
Jvion, Health Catalyst Support Diverse Use Cases but Fall Short on Performance; Clinithink NLP Capable but Not Fully Baked
Jvion, who by KLAS’ estimation has by far the largest client base in the healthcare AI market, also has the largest offering of prebuilt healthcare content for machine learning models/vectors. However, satisfaction is mediocre because clients feel outcomes are slower to come than Jvion led them to believe. Some customers cite implementation challenges, though they give Jvion credit for staying engaged. Implementation issues include not enough guidance from Jvion to help customers avoid common potholes and turn data into action. A few customers also feel Jvion needs to do more to help bolster clinician trust in AI technology.
Health Catalyst customers report highly diverse use cases; this is partly attributable to the way the vendor helps customers build models and is invested in driving outcomes. Compared to other measured vendors, Health Catalyst has the strongest healthcare expertise. Some clients feel the implementation takes too long and that outcomes are either early or will take a while to be realized.
Clinithink offers ready-to-use NLP algorithms for clinical notes and SNOMED. Clients praise the solution’s ability to process unstructured data. Clinithink is still building out their product portfolio from an NLP engine, so clients mention many features still need to be developed, resulting in lower satisfaction with overall product quality, needed functionality, and integration.
Other Validated Vendors: SAS AI Adoption Growing among Current Analytics Customers; Symphony AyasdiAI Healthcare Presence Shrinking
SAS: Well-known, cross-industry analytics vendor. Large healthcare presence in academic medical centers. In ML space, KLAS has validated clients using SAS tool to predict things like readmissions, avoidable ED visits, and no-shows. Adoption of AI capabilities growing among existing SAS customers.
Symphony AyasdiAI: Cross-industry ML platform vendor. Formerly Ayasdi; renamed when acquired by SymphonyAI Group in May 2019. Despite heavy background in the financial sector, all validated healthcare use cases are clinical. Most commonly, respondents use the product’s unsupervised learning capabilities (a fairly unique offering in healthcare AI today) for clinical variation management, disease management/prevention, and clinical research. Almost all customers interviewed for this research; customer base shrinking due to leadership turnover and lacking expertise and guidance to fully leverage tool.
ADDITIONAL INDUSTRY INSIGHTS
Types of AI Vendors
Measured in This Report
Purpose-Built AI Vendors:
Vendors primarily focused on analytics and AI. Offer and support dedicated, standalone product(s) designed specifically for AI. These solutions often include some level of prebuilt models, content, and uses cases specific to healthcare.
Analytics Platform Vendors with AI Infrastructure:
Analytics-focused vendors with AI infrastructure and/or platforms, typically used as a foundation to enable a do-it-yourself approach to AI (i.e., customers build their own models or use cases). These vendors’ technology is often used as the embedded AI foundation for other vendor products that are industry specific.
Not Measured in This Report
EHR Vendors with AI Capabilities:
EHR vendors who have developed and/or embedded AI models and platforms into their offerings. Mostly early in this process.
HIT Application Vendors with AI Capabilities:
Vendors who provide healthcare IT (HIT) applications that have AI capabilities but are not meant to be standalone AI applications. These vendors offer some level of AI in one or more products, typically embedded within a specific application—for example, a radiology system supported or enhanced by AI but not commercially sold as an AI product. Wide variation in what these vendors’ AI capabilities look like.
AI Adoption—From CHIME’s 2019 HealthCare’s Most Wired Report
As part of CHIME’s recent Most Wired survey, 483 provider organizations described their progress in adopting various types of AI solutions.
Validated Use Cases
The 57 organizations interviewed for this research report 37 distinct use cases across 11 categories within clinical, financial, and operational areas. While it is exciting to see AI being adopted across a wide variety of use cases, it is still too early to say whether these use cases can be scaled across broader customer bases.
AI Framework
The following framework shows which critical technologies, services offerings, and use cases KLAS has validated among healthcare organizations. (See page 3 for the definition of AI used in this report.)
Common Misconceptions about Healthcare AI
In this developing space, even organizations that have successfully achieved outcomes with AI technology experienced potholes along the way, often driven by misconceptions about healthcare AI and its implementation.
Misconception: Building the models is the most time-consuming AI task.
Reality: Don’t underestimate the time and effort it will take to prepare the data needed to test and build the models. Healthcare data is hard to clean and comes from many sources, and your organization may not have the expertise to feed the right variables or features into your models. Vendors and tools can help, but you need to do your own evaluation of the time and effort required to be successful with your models.
Misconception: Once the model is built, it will run itself.
Reality: Organizations must ensure long-term model applicability, accuracy, and performance. Healthcare data is often heterogenous; prebuilt models might work well in some cases, but you may have specific demographic situations to which they won’t apply, generating misleading results. Adopt and closely monitor model management to prevent concept drift, and encourage your vendor to do the same. You may be surprised at how much maintenance is required to keep your models viable over time.
Misconception: There are turnkey AI solutions that can drive outcomes.
Reality: There are plenty of user-friendly products that can help you build models quickly, and some vendors can provide deep data-science and healthcare expertise. But if you want tangible outcomes from the data, you need to consider operational aspects, like how many departments, resources, and facilities across the care continuum need to be involved. Getting buy-in from all disciplines before and after the implementation is critical. Without it, your model could end up as a shiny but useless algorithm.
Misconception: Our organization will jump at the chance to leverage AI tools.
Reality: Remember that culture shifts take time. It can be very challenging for staff (including clinicians) to trust AI-generated recommendations. Get staff buy-in early by demonstrating explainability and applicability and by sharing success stories.
How Healthcare Organizations Can Ensure Success
While technology is important, success with AI is perhaps even more dependent on an organization’s operations and change management. The following best practices come from some of the industry’s most successful AI users.
Embed AI in the workflow:
When creating models, observe clinicians’ workflows and find the appropriate places in which to embed models or insights so that they are located within users’ regular routines and are not disruptive. Promote AI insights to clinicians as extra information to act on, not extra hoops to jump through.
Bring together experts on AI, data science, modeling, analytics, and subject matter:
Promote interdisciplinary collaboration. An AI project cannot be successfully rolled out unless all groups work closely together.
Take ownership for driving change management and operationalizing insights:
Take a social engineering approach to get staff engaged in implementing changes. Report progress and successes to staff to encourage adoption.
How Vendors Can Help Drive Client Success
For healthcare organizations to be successful with their AI solutions, they need vendors that do much more than deliver a high-quality, technologically capable product. The best practices below were reported by vendors’ most successful AI customers.
Deliver comprehensive services for AI platform:
Through AI support services, some vendors offer resources like client success managers, dedicated data scientists, and field engineers to ensure models are built and deployed successfully and in a timely fashion. Customers benefit when vendors have healthcare experts on staff acting as these resources. Clients appreciate when vendors can evaluate an organization’s current AI state and provide support accordingly.
Provide strong customer training:
Effective training may come through a formal training program, tailored on-site training, a certification program, training material, and/or continuing access for questions and ongoing learning. Customers are most satisfied when their vendor trains the organization’s analysts to give them the data science expertise needed to build, deploy, and maintain their own models.
Be a humble, active partner:
Healthcare AI is still a very young market, and all vendor companies are experiencing growing pains and learning as they go. Clients need vendors who are transparent about their journey and acknowledge their need for customer partners in development. In this partnership, vendors learn best when they are responsive to constructive feedback and then correct course.
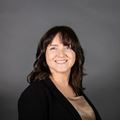
Writer
Amanda Wind

Designer
Jess Wallace-Simpson
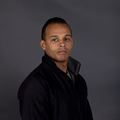
Project Manager
Robert Ellis
This material is copyrighted. Any organization gaining unauthorized access to this report will be liable to compensate KLAS for the full retail price. Please see the KLAS DATA USE POLICY for information regarding use of this report. © 2025 KLAS Research, LLC. All Rights Reserved. NOTE: Performance scores may change significantly when including newly interviewed provider organizations, especially when added to a smaller sample size like in emerging markets with a small number of live clients. The findings presented are not meant to be conclusive data for an entire client base.