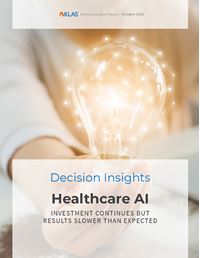
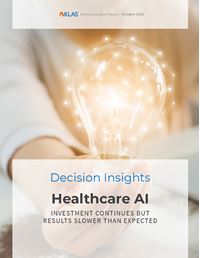
Healthcare AI 2020
Investment Continues but Results Slower Than Expected (A Decision Insights Report)
The benefits of integrating artificial intelligence (AI) into the healthcare ecosystem are undeniable,† and investment from healthcare organizations is increasing at a fast pace. Yet few organizations have settled on any one AI vendor as their go-forward choice—most use a hodgepodge of solutions to meet their needs. In this report, KLAS examines the recent AI purchase decisions of 47 organizations to determine which vendors are being considered and chosen, which are being replaced, and why provider and payer organizations choose the AI solutions they do (technology? service? healthcare expertise?). Additionally, we share customer satisfaction data from current users of ClosedLoop.ai, DataRobot, Epic, Health Catalyst, Jvion, and KenSci and examine healthcare’s perceptions of the cross-industry AI giants—Amazon, Google, IBM, and Microsoft.
† For details about how KLAS defines AI in healthcare, see "Healthcare AI 2019: Actualizing the Potential of Artificial Intelligence."
Jvion Has High Market Visibility & Purchase Consideration, Struggles to Deliver; Epic Growing Fast, with Embedded Workflows an Advantage
One of the earliest companies to the healthcare AI market, Jvion has high visibility and one of the largest client bases. Potential customers are drawn to Jvion’s technology, expertise, healthcare-specific vectors, and ability to prescriptively suggest interventions to improve patient outcomes. Satisfaction among current clients has declined since last year, with nearly a quarter of respondents reporting overall dissatisfaction. These clients say that despite excellent efforts from Jvion executives, it has taken longer than expected to achieve results and has been challenging to realize an ROI.
Epic Cognitive Computing is the most widely adopted EMR AI solution and the only one with enough live customers for satisfaction data to be shared. Used solely by Epic EMR customers, it is often selected for its workflow integration and easy access to EMR data. Epic’s AI models are used most heavily in clinical areas, with readmission and sepsis prediction the most frequently reported use cases. Still maturing, the solution may not be a good option for Epic organizations that aren’t prepared to operationalize models and drive outcomes on their own. Customers feel implementations and support have improved in response to their feedback, and all plan to keep the solution long term.
Fewer than 10 customers (most in pilot phases) are live with HealtheDataLab, Cerner’s machine learning (ML) platform, which customers can use to build and deploy their own algorithms (the platform does not come with prebuilt models). The data is fed through HealtheIntent. The platform is still early and receives little consideration. Those who do look at it are mostly Cerner EMR customers—they report interest in the ability to customize their own models and ingest non-Cerner data.
For more information on the AI offerings of EMR vendors, please see ”Healthcare EMR AI 2020: A Closer Look at EMR Offerings.”
KenSci Client Satisfaction Remains High; Health Catalyst and DataRobot See Decrease
KenSci, DataRobot, and Health Catalyst all offer ML platforms that enable customers to produce their own algorithms and models; KenSci also offers healthcare-specific models in clinical and operational areas. Customer satisfaction for all three vendors comes from a limited sampling of customers.
Organizations that considered KenSci cite the vendor’s AI expertise, partnership, and healthcare-specific models as well as the flexibility to build their own models as key factors in their consideration. Those who ended up selecting other vendors felt KenSci wasn’t mature enough or lacked specific functionality they needed. Existing clients are overall very satisfied, although several note that both they and KenSci are still in the learning stage. They are optimistic about the future and look forward to receiving new dashboard functionality and expanding adoption within their organizations.
Potential DataRobot customers point to the vendor’s partnership and tools (e.g., automated ML platform, API capabilities, and ease of use) as strengths. A few feel DataRobot lacks some functionality an end-to-end platform should provide. Most current customers are satisfied, highlighting DataRobot’s training, technical support, and expertise. Satisfaction has dropped over the last year, due mainly to the platform’s incompleteness, which costs customers time and manual work for things like data preparation, model production and deployment, and performance improvement. Customers also report concern about DataRobot’s lack of healthcare expertise.
Health Catalyst is transitioning their healthcare.ai product from being free, open-source R/Python software to offering more comprehensive data science services and embedded data science software. Feedback in this report comes from customers of both the free software and the software-plus-services offering. Satisfaction among current customers is weak, with four of seven respondents reporting dissatisfaction (overall score below 70). These customers appreciate the vendor relationship but describe the product as immature and requiring significant co-development effort. Even satisfied clients are looking for more AI capabilities and a better ROI.
Expertise and Partnership Top Decision Drivers, as Exemplified by Newcomer ClosedLoop.ai
The top factor driving purchase decisions in healthcare AI is expertise (i.e., healthcare-specific knowledge as well as ML and data science expertise). Offering a data science/ML platform and catalog of prebuilt models, healthcare-dedicated newcomer ClosedLoop.ai focuses on population health management, risk adjustment, and quality improvement. Though considered in just six decisions (13%), the vendor was selected in five. These customers cite strong healthcare expertise and ClosedLoop’s ML Content Library (which includes customizable model templates and resusable predictive features) as significant factors in their decision. Many clients highlight the fact that the predictive features are explainable, which makes for easier end-user adoption. All current customers interviewed are satisfied or highly satisfied, reporting strong partnership, collaboration, and responsiveness. Some would like better training. Consideration of Medial EarlySign, another healthcare-dedicated vendor with strong expertise, is on the rise. The vendor offers consulting services, a library of prebuilt content (AlgoMarkers), pattern-recognition algorithms that evaluate patient data and determine disease risk, and predictive and prescriptive data outputs to help influence decision-making. Medial EarlySign’s client base is small, and while customer satisfaction data is currently too limited for specific details to be shared, the three interviewed customers had only positive feedback to report.
Cross-Industry AI Giants Perceived as Average Overall—Microsoft Seen as the Strongest, While IBM Trails
The large cross-industry AI giants—Amazon, Google, IBM, and Microsoft—are growing their AI investments and partnerships in healthcare, generating increased interest from payer and provider organizations. Of the four, IBM receives the most considerations but is the only one not actually selected in any of the 47 purchase decisions included in this research—organizations passed on IBM due to skepticism about outcomes as well as concerns about cost and over-marketing.
Despite the increased interest in these vendors, customer satisfaction data is currently limited. Therefore, KLAS gathered perceptions from payer and provider organizations—some customers, some not—on the strength of each vendor’s AI solution. Some respondents felt it was too early even to share perceptions, but those that could reported average strength overall—in general, organizations are intrigued by the vendors’ AI technology and expertise but worry about their relative inexperience in healthcare, the fact that their solutions reside in the cloud (an area that healthcare organizations are just getting into), and the burden of integrating the tools into workflows.
Half of respondents who shared perceptions of Microsoft feel the vendor has a strong healthcare AI offering. Highlighting Microsoft’s healthcare partnerships (e.g., Microsoft Azure is leveraged by Epic, Health Catalyst, Jvion, and KenSci), organizations feel Microsoft has more healthcare expertise than the other cross-industry vendors. Azure’s AI infrastructure and platform are integrated with other highly adopted Microsoft products, such as Office 365 and Teams, and respondents note that Microsoft seems to have better security and a strong data-protection guarantee. Respondents with less positive perceptions report slow responses, a complicated contracting process, and a tendency to overpromise and underdeliver.
Google is noted for advanced capabilities that enable customers to create and develop core algorithms capable of impacting patient outcomes, but they are not viewed as having a strong platform. Google also stands out for the quantity of open-source information they have created that is used in data science. However, Google is less well known in healthcare, and respondents feel they would have trouble trusting Google with their data.
Amazon is gaining mindshare in healthcare, and adoption of their AWS cloud services—into which Comprehend, SageMaker, and other AI tools are integrated—is on the rise. Amazon has a track record of success and a solid reputation of being innovative and adaptive but is viewed as lacking a nuanced understanding of healthcare. Additionally, respondents are unclear what Amazon’s healthcare and AI development strategies are, and there is skepticism about Amazon’s ability to integrate operationally.
IBM Watson Health—one of the more widely known AI technologies in healthcare—has the most comprehensive store of indexed medical publications in the industry and offers NLP for extracting drug-database prescription information. IBM has had a longer history in healthcare AI; however, real outcomes have been slow to materialize in this time frame, contributing to almost half of respondents viewing IBM’s AI offerings as weak. These respondents feel IBM’s marketing outpaces their delivery. Many respondents who have worked with IBM in the past report that good technology and sometimes-satisfactory results are dampened by overpromising, insufficient support, or low value.
Healthcare Mindshare Low for Other Cross-Industry AI Vendors
Other cross-industry AI vendors like Dataiku, dotData, H20.ai, SAS, and Symphony AyasdiAI have high mindshare in other industries but seem to lack traction in healthcare. While payer and provider organizations are often aware of these vendors, they do not frequently consider them in AI purchase decisions (see purchase decisions chart on previous page).
AI in Imaging: Where Is the Market Going?
Imaging is a critical AI and ML use case in healthcare, and vendors and healthcare organizations alike are focusing increased attention on AI that can improve diagnosis processes, develop treatment protocols, and personalize patient care, among other use cases. In March 2020, KLAS published research on imaging AI perceptions; that report explored healthcare organizations’ plans for growth in this area as well as their perceptions of which vendors would be best positioned to help. We now have very early data on three purpose-built, FDA-approved imaging AI vendors who are emerging as early leaders and are popping up in KLAS’ Decision Insights data—Aidoc, Viz.ai, and Zebra Medical Vision.
Founded in 2016, Aidoc is perceived as a fast mover who is ahead of the game with multiple algorithms that have been FDA approved and adopted by a wide range of healthcare organizations, including teleradiology providers, imaging centers, inpatient hospitals, and outpatient clinics. Customers say the Aidoc solution is vendor agnostic and embedded in their PACS and workflows. Aidoc’s vendor partners include Agfa HealthCare, Change Healthcare, Fujifilm, GE Healthcare, Medicalis, MModal, Nuance, Philips, Sectra, and Visage Imaging.
Viz.ai’s FDA-approved stroke algorithm uses machine learning to quickly process images and diagnose stroke patients. The vendor’s platform is mobile enabled, automating workflows for clinician users (who—uniquely—are the primary decision drivers in purchases of Viz.ai). Multiple customers that chose Viz.ai over RapidAI (another AI firm focused on stroke care) felt Viz.ai had better customizability, a wider variety of modules, and a more integrated communication platform. The limited amount of customer satisfaction feedback is positive—customers are satisfied with the results they have achieved and with Viz.ai’s partner approach and easy-to-use product. The vendor partners with Medtronic.
Zebra Medical Vision’s all-in-one AI platform has multiple FDA-approved algorithms, and their customer base encompasses both small and large customers, including hospitals, radiology groups, and imaging centers. Use cases for Zebra’s imaging analytics engine include brain, lung, liver, cardiovascular, and breast imaging as well as x-rays (multiple types of conditions). Customers report confidence in Zebra and note the vendor’s traction in the market. Zebra is seen as nimble and willing to partner with customers and other vendors, including Amazon, Change Healthcare, Google, Johnson & Johnson, Intelerad, Mach7 Technologies, Nuance, and Philips.
Insights on the AI Purchase Decision Process
AI technology purchases are most often driven by CIOs, other IT leaders, and clinical leaders (including chief quality officers, CMIOs, CMOs, CMQOs, and CNOs). Often, clinical, technology, and analytics/data science leaders work together to figure out how AI can help them solve real patient problems. However, decisions are rarely made by just one or two stakeholders—they are generally vetted by a multidisciplinary team of leaders.
Insights on Enterprise-Wide AI Plans
Most respondents (64%) plan to or would like to use their AI solutions enterprise-wide (i.e., in clinical, financial, and operational areas). Most of the 36% who don’t expect to use their AI solution enterprise-wide purchased their solution for very specific areas or use cases; others don’t currently have all the needed data loaded in the AI solution or would like to better deploy current models before expanding. The 24% who would like to use their AI solution across the enterprise but don’t plan to state that they would like to achieve higher adoption of and better outcomes with their current use cases before applying AI to other areas.
Vendor Summaries
Most widely adopted AI solution from EMR vendor, and only EMR AI solution with enough live customers for satisfaction data to be shared. Considered in 26% of decisions, Epic is often selected for workflow integration and ease of access to EMR data. Customers are still relatively new, having used the product on average a little less than three years. AI models used most heavily in clinical areas, with readmission and sepsis prediction the most frequent use cases. Operational use cases mostly involve bed planning and no-show prediction.
Solution is still maturing but considered a good option by Epic EMR customers who are prepared to operationalize models and drive outcomes themselves. Not seen as most advanced AI product, but customers say it is improving quickly, as other Epic products have, and all plan to keep the solution long term. Implementations and support have improved in response to customer feedback.
+
“Our general experience with Epic was that it took weeks to get models set up rather than months like it did with another vendor that we worked with. Also, Epic’s system was much less costly than the other vendor’s system. Epic has basically all the important models that we want to use, so there is no reason to work with another vendor.” —CMIO, recently selected Epic
–
“Epic doesn’t do a great job of defining what Cogito is. . . . We never really know what falls under a product’s umbrella. I have lost confidence that the vendor can deliver everything I need. If there is something that Epic has that works, I am certainly going to look at it first, but they don’t always have what I need. Epic doesn’t have the bandwidth to custom build things for me. If they do build something, I need it to work. If it works, I have to fit it into our workflow. The vendor’s track record of passing all three of those steps is not great. But if Epic had something, I would look at it first because it would involve the least amount of pain and configuration on my end. But no one wants a race car with three wheels.” —CMIO, current customer
As one of the earliest healthcare AI companies, has high visibility/prominence and one of the largest market shares in healthcare AI. Also has highest mindshare, being considered in 32% of decisions. Often considered due to technology and expertise and also to references from other healthcare organizations. Respondents appreciate Jvion’s breadth of healthcare-specific vectors and ability to prescriptively suggest interventions. Overall satisfaction among current clients has decreased since last year, and nearly a quarter of respondents are unsatisfied, saying it has been challenging to realize an ROI, despite excellent efforts from Jvion executives. For some, results have taken longer than expected to achieve, and the solution has ended up being costly.
+
“Jvion is on the cutting edge and is innovative. . . . Jvion is one of the prominent companies out there. . . . Jvion stood out because of prior experience that other leaders at our system had had with Jvion at other organizations. Jvion made a very strong sales pitch, particularly to some of our C-level executives. So many systems of our same size have been picking up on Jvion and other large and emerging AI companies. Jvion has a very high level of visibility.” —Director, recently selected Jvion
–
“We had more of a vendor relationship with Jvion than a partnership. Overall, we just didn’t see the results we were expecting. . . . We expected to get a certain benefit, but we didn’t receive it based on how expensive it was to maintain Jvion as our vendor. We thought we were going to get a certain level of expertise, and that level just wasn’t reached. . . . Internally, we had enough resources that we could have done the same thing without them.” —Manager, replacing Jvion
Newcomer, healthcare-dedicated AI company; offers data science/ML platform as well as prebuilt model catalogue focused on population health management, risk adjustment, and quality improvement. Has small but growing base among payers, providers, and ACOs. Offers healthcare-specific support for data normalization, feature engineering, automated model training, model deployment, and report generation. First-time KLAS measurement. Considered in just six validated decisions (13%) but selected in five. Organizations that chose them cite strong healthcare expertise and ClosedLoop’s ML Content Library, which includes customizable model templates and reusable predictive features and improves patient outcomes and patient retention. Many highlight that these features are explainable, making for easier adoption.
All six interviewed current customers are satisfied/highly satisfied, reporting strong partnership, collaboration, and responsiveness. Some would like better training. The organization that considered but did not choose ClosedLoop.ai was looking for broader operational and financial use cases/capabilities.
+
“ClosedLoop.ai wasn’t trying to fit a square peg into a round hole. They understood that we had a unique interest and a unique situation. ClosedLoop.ai’s healthcare expertise was a big deal to us. . . . It didn’t take a lot to translate things, and they adapted their approach to create a predictive model for our unique interest in the data streams.” —CEO, recently selected ClosedLoop.ai
–
“We were looking for something not so healthcare driven because we have multiple business lines. We have an operations and marketing team that wants to use AI that is not necessarily specific to healthcare. Our marketing team cares about clinical-based outcomes but is looking for customer-acquisition models. We also have a line of business for our insurance vertical, so we need to be able to do modeling similar to what the insurance and finance folks are doing. ClosedLoop.ai was capable but is solely focused on healthcare and health outcomes.” —Data science director, considered but did not select ClosedLoop.ai
Cross-industry, automated ML platform. As purpose-built AI platform, solution is use-case agnostic, enabling clients to create own models. Acquisition of Paxata, a data prep and blend company, enables customers to load, clean, and manipulate data without having to use other data-cleansing tools. Considered in 21% of decisions. These organizations highlight tools (automated ML platform, API capabilities, ease of use) and partnership; a few feel DataRobot lacks some functionality an end-to-end platform should provide (can’t do building, modeling, displaying, iterating all in one platform).
Despite four-point drop in overall score since last year, most of the six interviewed clients are satisfied, highlighting support as a strength. They like that their own data scientists have access to helpful DataRobot resources. Ease of use is also a strength, and solution is described as intuitive. Drop in satisfaction is due to platform’s incompleteness, which costs customers time and manual work for things like data preparation, model deployment and production, and performance improvement. Additionally, some respondents feel the company lacks healthcare-specific knowledge.
+
“DataRobot came in, and our experience was pretty good. They came in, helped us, and basically gave us as much time as we needed to evaluate the product. The vendor essentially gave us unlimited processing power, and that was huge.” —Manager, recently selected DataRobot
–
“DataRobot has a platform-based approach. I think pretty highly about the way they allow customers to optimize models, but DataRobot’s feature set is not complete. The real problem in AI and data modeling is that people who know a lot about healthcare processes and clinical care are very far away from the people that actually do data modeling and analytics. We decided to go with a system that allows us to go from building, to modeling, to displaying, to iterating all in one platform.” —CMIO, considered but did not select DataRobot
healthcare.ai product is transitioning from free, open-source R/Python software to more comprehensive data science services and embedded data science software. Free software continues to be supported and maintained. The broader services-plus-software offering supports clients’ change-management and workflow-optimization efforts and sometimes includes building and deploying predictive models. Considered in 19% of decisions, Health Catalyst is often noted for strong partnership. Satisfaction among current customers (including users of both the free software and the broader offering) is poor, with 4 of 7 respondents reporting dissatisfaction (overall score below 70). These customers appreciate the vendor relationship but describe the product as immature and requiring significant co-development effort. Additionally, some report staff turnover with no transition plan. Even satisfied clients are looking for more AI capabilities/maturity and more ROI.
+
“I am interested in learning more about Health Catalyst. I love what they do. I love the white papers that they put all over the internet in terms of the successes and how people use data to change care. . . . Health Catalyst holds the customer’s hand and helps on the operational side. So their customers not only get a model but also get Health Catalyst’s knowledge and firepower to implement the model. Other vendors don’t offer that.” —CMIO, considering Health Catalyst
–
“Health Catalyst is the best vendor we have ever worked with. Having said that, their AI capabilities are still pretty early. The one that we have used the most is their model for COVID-19 forecasting that they stood up on their website. That is somewhat useful, although there are a lot of problems that it created for the end users where the prediction forecast was just difficult for them to process and think about.” —CMIO, current customer
Healthcare-specific platform reliant on Microsoft technology and focused on explainable AI. Serves some payers, but most clients are large, complex health systems that derive models from KenSci’s platform and choose KenSci for their enterprise AI projects. Considered in 17% of decisions. Those who choose KenSci cite the vendor’s AI expertise, partnership, and healthcare-specific models as well as the flexibility to build their own models. Those who didn’t end up selecting KenSci feel the company isn’t mature enough or doesn’t have specific functionality they need. Current clients are very satisfied, although several note that both they and KenSci are still in the learning stage. They are optimistic about the future and look forward to new dashboard functionality and expanding adoption within their organizations. Several highlighted the honesty and upfront communication of KenSci’s leadership. One CIO said it took longer than anticipated to build and tune the models.
+
“We chose KenSci for a variety of reasons. They were experienced, and we liked that they really leveraged Microsoft Azure. They were up and coming, and they had some healthcare models already developed. KenSci had a good price point. We did do an RFP, and we looked at a number of vendors. At the time, there weren’t a lot of vendors out there.” —CIO, recently selected KenSci
–
“We looked at a company called KenSci. KenSci had the capability of monitoring AI tools and technology. We were worried that more models would be developed and the AI tool would get replaced. We were worried about the monitoring capabilities. We had conversations, and then things dropped off. The company wasn’t mature enough.” —Director, considered but did not select KenSci
About This Report
Data for this report comes from three sources: (1) KLAS performance data, (2) KLAS perception data, and (3) KLAS Decision Insights data.
KLAS Performance Data
Each year, KLAS interviews thousands of healthcare professionals about the IT products and services their organizations use. These interviews are conducted using a standard quantitative evaluation, and the scores and commentary collected are shared online and in reports like this one so that other providers and IT professionals can benefit from their peers’ experiences. To enable readers to more quickly understand high-level differences in vendor performance and give better context as to how each product compares to other offerings in the market, KLAS has organized the questions from the standard evaluation into six customer experience pillars—culture, loyalty, operations, product, relationship, and value.
The performance data in this report was collected over the last 12 months; the number of unique responding organizations for each solution is given in the chart below.
What Does “Limited Data” Mean?
Some products are used in only a small number of facilities, some vendors are resistant to providing client lists, and some respondents choose not to answer particular questions. Thus a vendor’s sample size may vary from question to question and may not reach KLAS’ required threshold of 15 unique respondents. When a vendor’s sample size for a particular question is less than 15, the score for that question is marked with an asterisk (*) or otherwise designated as “limited data.” If the sample size is less than 6, no score is shown. Note that when a vendor has a low number of reporting sites, the possibility exists for KLAS scores to change significantly as new surveys are collected.
Overall scores are measured on a 100-point scale and represent the weighted average of several yes/no questions as well as other questions scored on a 9-point scale.
KLAS Perception Data
To supplement the data gathered with the standard evaluation, KLAS also creates various supplemental evaluations that delve deeper into the most pressing questions facing healthcare technology today. For this AI research, KLAS used a supplemental evaluation to gather deeper insights from provider and payer organizations regarding their purchase-decision process, plans for enterprise AI use, and perceptions of the large, cross-industry AI giants (i.e., Amazon, Google, IBM, and Microsoft).
KLAS Decision Insights Data
All references in this report to organizations’ purchasing motivations come from KLAS’ Decision Insights data. Since 2017, KLAS has been gathering information as to which vendors are being replaced, considered, and purchased and what factors drive these decisions. KLAS Decision Insights data does not represent a comprehensive census or win/loss market share study. Rather, it is intended to help provider and payer organizations understand which vendors have market energy and why. The Decision Insights data in this report is based on 47 AI-technology purchase decisions.
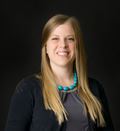
Writer
Elizabeth Pew

Designer
Jess Wallace-Simpson
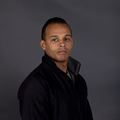
Project Manager
Robert Ellis
This material is copyrighted. Any organization gaining unauthorized access to this report will be liable to compensate KLAS for the full retail price. Please see the KLAS DATA USE POLICY for information regarding use of this report. © 2024 KLAS Research, LLC. All Rights Reserved. NOTE: Performance scores may change significantly when including newly interviewed provider organizations, especially when added to a smaller sample size like in emerging markets with a small number of live clients. The findings presented are not meant to be conclusive data for an entire client base.