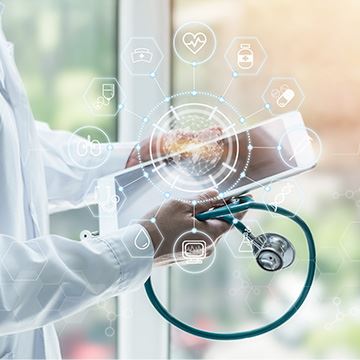
AI in Healthcare: Organizations Starting to Expand beyond Clinical and PHM Use Cases
Artificial intelligence has dominated headlines in recent years as understanding of current capabilities and future promise has grown. The technology’s use cases are many and varied, with the potential to impact many facets of business and life, including healthcare. How is AI currently being used by provider and payer organizations, what outcomes have they achieved, and what do they have planned for the future? A recent report by KLAS—titled Healthcare AI 2024: Use Cases Expanding to Meet New Market Needs—sheds light on these and other AI questions.
Use of AI in Healthcare Is Quickly Changing
Respondents interviewed for the report say that their use of AI is evolving. For starters, in response to the staffing shortages, budget constraints, and other difficulties of the post-pandemic landscape, more healthcare organizations are adopting data science platforms and other AI solutions.
On top of that, organizations that already use AI are expanding their strategies to encompass a wider variety of use cases. In the past, adoption of AI in healthcare centered mostly on clinical and population health management (PHM) use cases. This trend can be seen in the chart below. However, given the challenges of today’s environment, there is a growing focus on using AI to increase operational efficiency. Alongside these operational efforts, interviewed organizations also report an increase in use of AI in financial areas. In total, 50% of respondents say they are expanding the use of their AI solution into additional areas.
Majority of Reported Outcomes Still Focus on Clinical and PHM Areas
When it comes to actual achieved outcomes, the majority of respondents still largely report results related to various value-based care and population health management activities. As a chief data and analytics officer reported, “The primary return on investment from the product is being more proactive in our intervention so that we can manage our population more effectively. We know the risk a bit better and use risk stratification insight for care management and outreach. Also, the product provides a feature library that accelerates the development of the predictive models. If we were to do that from scratch using traditional tools, developing the models and doing life cycle management would take longer. We are getting value from the accelerated delivery.”
Some of the clinical and PHM-related outcomes reported by respondents include the following:
- Identification of high-risk members for care management
- Increased quality measures
- Higher-than-expected outreach numbers
- Improved risk capture
- Improved marketing campaigns
- More-creative strategies for approaching underprivileged populations
- Substance abuse prediction
- Improved denials management
Time will tell what outcomes organizations might be able to achieve from their new focus on operational and financial use cases.
Vendor Support Crucial to Success
In addition to reporting on use cases and adoption, the report examines customer satisfaction with data science platforms and AI solutions from five key vendors—ClosedLoop, Epic, Health Catalyst, N1 Health, and Oracle Health. As shown in the chart above, satisfaction with the various offerings varies. Given the inherent complexity of the technology, it is no surprise that the quality of the vendor service and support is a key contributing factor in customer satisfaction and can make or break the customer experience. Indeed, the vendors who perform better in this space—including ClosedLoop, Epic, and N1 Health—are those who provide a higher level of support and guidance to their customers. More details about how each of the vendors performs in this and other key areas—such as outcomes and ease of use—can be found in the report.
Other Key Market Trends
We’d like to finish this overview of the report by mentioning some of the other notable trends KLAS is seeing. The research also revealed the following about the healthcare AI market:
- Larger organizations (i.e., organizations with over 500 beds) are the most likely to use a data science platform.
- Most interviewed organizations report that their solution is used and monitored by data scientists.
- AI technology from EHR vendors is the most common type of AI solution in use by provider organizations. This technology is supplemented by third-party or homegrown solutions as necessary.
- Most of the organizations that use their EHR vendor’s data science platform use the solution’s prebuilt models rather than building their own custom models.
- Workflow integration is crucial to success. Many respondents highlight the importance of training and note that data must be actionable and embedded within user workflows. Organizations continue to want guidance and partnership from their vendors to help them develop an AI strategy and encourage end-user adoption.
© Chinnapong / Adobe Stock